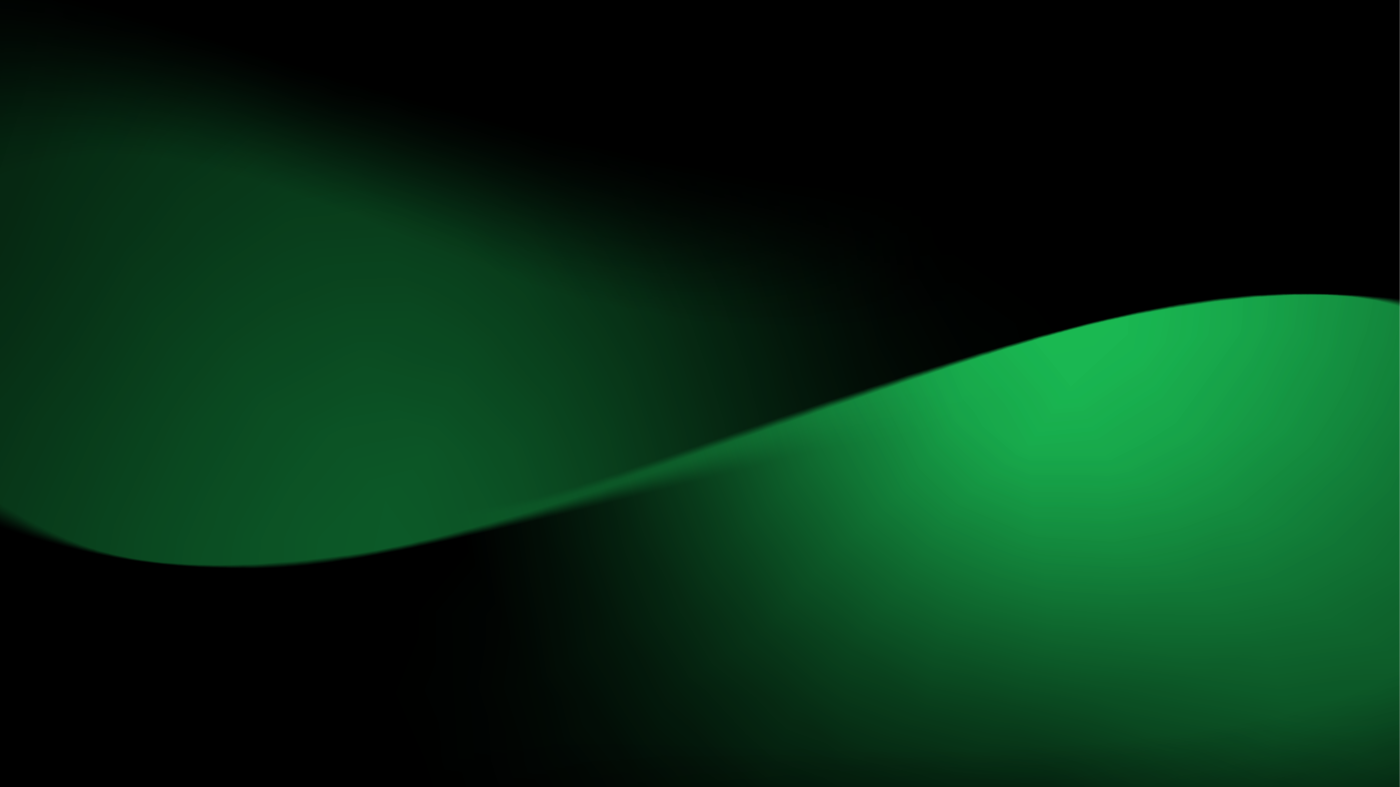
News
Deep Bio Unveils Research Findings on Performance Evaluation of AI Algorithm for Breast Cancer Analysis
May 21, 2024
Deep Bio utilizes deep learning-based algorithms to differentiate breast cancer lesions accurately, optimizing diagnostic accuracy
SEOUL, SOUTH KOREA, May 21, 2024
Deep Bio today announced the publication of a study evaluating the performance of its AI algorithm for breast cancer analysis to accurately differentiate between invasive ductal carcinoma (IDC) lesions from non-invasive ductal carcinoma in situ (DCIS) lesions in biopsies taken after breast cancer surgery.
The research, conducted in collaboration with the Catholic University of Korea Bucheon St. Mary’s Hospital and Korea University Guro Hospital, has been published in a special issue of MDPI Bioengineering titled “Computational Pathology and Artificial Intelligence.”
Breast cancer is the most prevalent cancer among women worldwide, accounting for 24.5% of all female cancers, with a mortality rate of 15.5%, the highest among women’s cancers. Pathologically, breast cancer is categorized into various types, with invasive ductal carcinoma (IDC) being the most common, constituting 70-80% of all cases. Accurate differentiation between IDC and ductal carcinoma in situ (DCIS) is critical for effective treatment planning. The “2022 Korean Breast Cancer White Paper” published by the Korean Breast Cancer Society, reported 24,933 IDC and 4,816 DCIS cases in South Korea in 2019, underscoring the need for precise diagnostic tools. Predicting the characteristics, size, and severity of lesions in invasive ductal carcinoma (IDC) and non-invasive ductal carcinoma (DCIS) accurately through this study indicates a significant advancement.
Traditional pathology involves examining cancer cells’ growth patterns and histological characteristics using a microscope. However, mixed patterns of IDC and DCIS within the same lesion can complicate manual assessment and prognosis prediction. This is where AI-powered algorithms can revolutionize diagnostics.
Specifically, Deep Bio’s Multi-resolution Selective Segmentation Model for Breast Cancer (MurSS) addresses these challenges by analyzing hematoxylin and eosin (H&E) stained breast cancer pathology slide images to segment breast cancer lesions automatically. This model enhances diagnostic accuracy by leveraging multi-resolution images and introduces a selective segmentation method to automatically exclude uncertain areas from learning, thereby increasing the stability and reliability of model results.
MurSS achieved a pixel-level accuracy of 96.88% (95% confidence interval 95.67% to 97.61%) on breast cancer H&E slides, outperforming existing deep learning models.
CTO Tae-Yeong Kwak of Deep Bio said, “Using the Multi-resolution Selective Segmentation Model (MurSS), we can more accurately measure cancer areas on whole slide image of breast tissues, aiding in the measurement of invasive cancer area by excluding in situ carcinomas,” he added. “I hope our AI algorithm for breast cancer enables pathologists to identify precise cancer lesions by improving predictions with automated cancer marker analysis.”
Deep Bio continues to innovate with AI solutions for breast cancer analysis. DeepDx® Breast – Resection is a screening solution that automatically detects areas of interest in breast excision slide images, while DeepDx® Breast – SLNB (Sentinel Lymph Node Biopsy) has maintained its top performance since securing first place in the Camelyon17 Challenge in 2019, a global image analysis competition focused on breast cancer lymph node metastasis detection.
About Deep Bio
Deep Bio is an AI healthcare company dedicated to advancing the field of cancer pathology. Focusing on deep learning, the company develops cutting-edge In Vitro Diagnostic Software as Medical Devices (IVD SaMDs) to empower pathologists and medical professionals with state-of-the-art tools for more accurate cancer diagnosis and prognosis.
For more information, visit the website: www.deepbio.co.kr.